Amazon Web Services (AWS) Kinesis is a cloud-based service that can fully manage large distributed data streams in real-time. This serverless data service captures, processes, and stores large amounts of data. It is a functional and secure global cloud platform with millions of customers from nearly every industry. Companies from Comcast to the Hearst Corporation are using AWS Kinesis.
Key takeaways
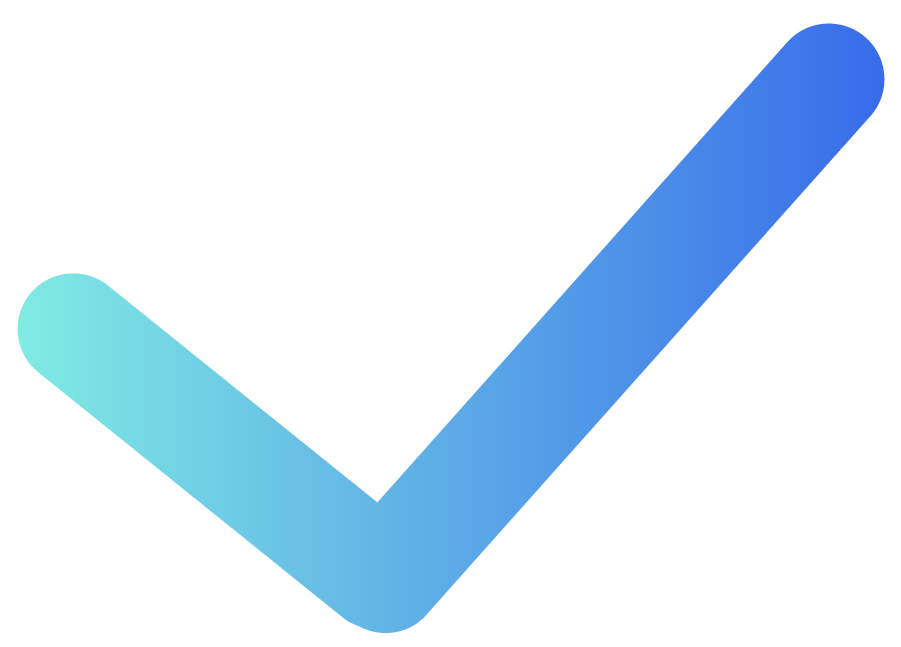
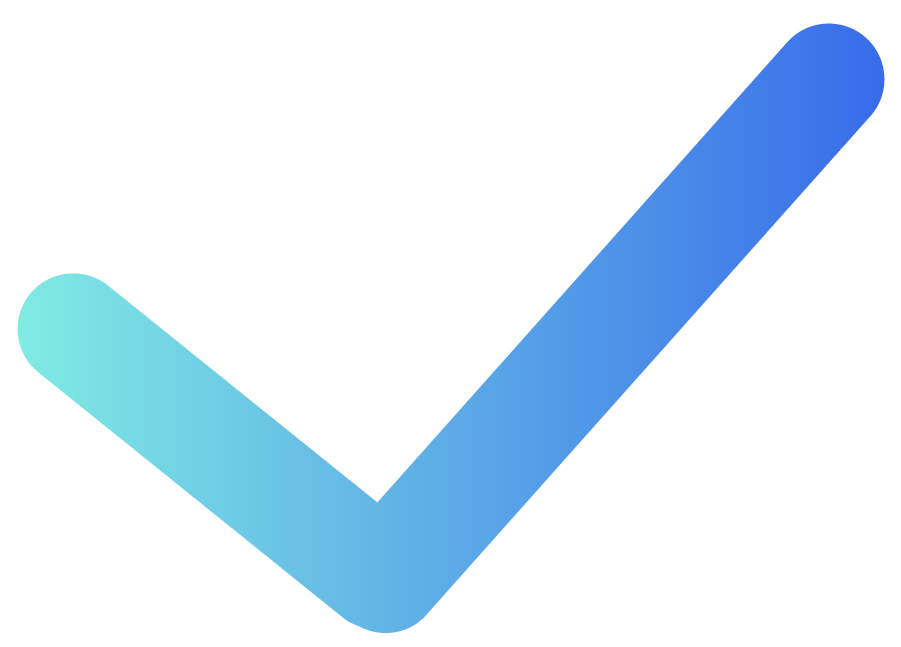
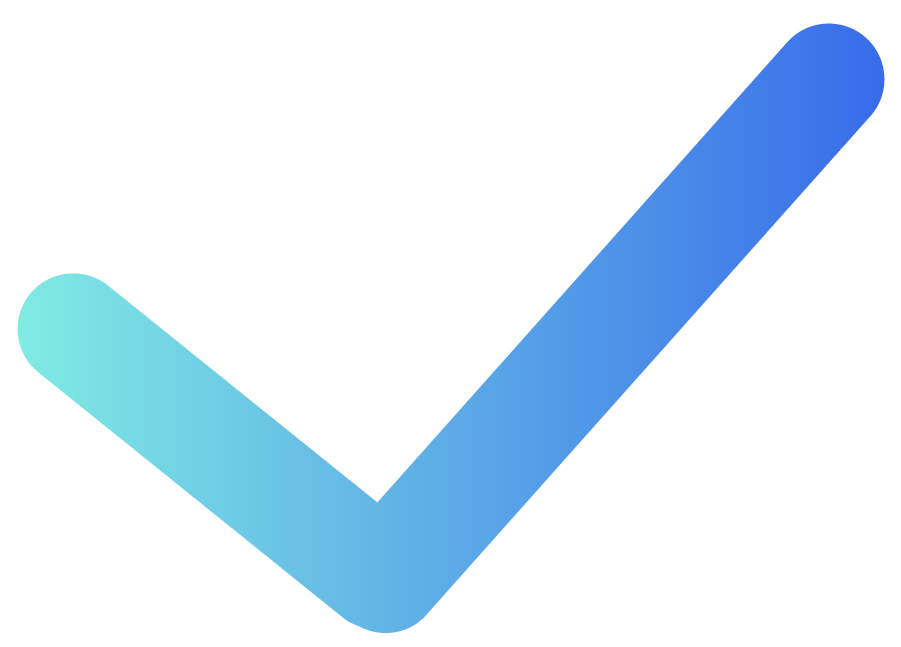
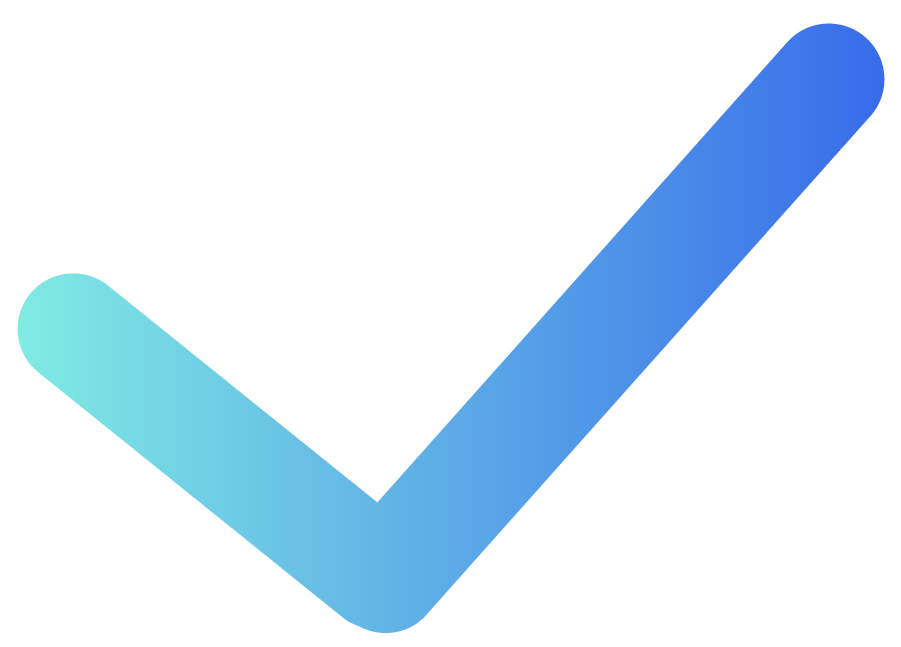
What is AWS Kinesis?
AWS Kinesis is a real-time data streaming platform that enables businesses to collect, process, and analyze vast amounts of data from multiple sources. As a fully managed, serverless service, Kinesis allows organizations to build scalable and secure data pipelines for a variety of use cases, from video streaming to advanced analytics.
The platform comprises four key components, each tailored to specific needs: Kinesis Data Streams, for real-time ingestion and custom processing; Kinesis Data Firehose, for automated data delivery and transformation; Kinesis Video Streams, for secure video data streaming; and Kinesis Data Analytics, for real-time data analysis and actionable insights. Together, these services empower users to handle complex data workflows with efficiency and precision.
WS Kinesis transforms massive data streams into actionable insights in real time, empowering businesses to make smarter, faster decisions.
To help you quickly understand the core functionality and applications of each component, the following table provides a side-by-side comparison of AWS Kinesis services:
Feature | Video streams | Data firehose | Data streams | Data analytics |
What it does | Streams video securely for storage, playback, and analytics | Automates data delivery, transformation, and compression | Ingests and processes real-time data with low latency and scalability | Provides real-time data transformation and actionable insights |
How it works | Uses AWS Management Console for setup; streams video securely with WebRTC and APIs | Connects to AWS and external destinations; transforms data into formats like Parquet and JSON | Utilizes shards for data partitioning and storage; integrates with AWS services like Lambda and EMR | Uses open-source tools like Apache Flink for real-time data streaming and advanced processing |
Key use cases | Smart homes, surveillance, real-time video analytics for AI/ML | Log archiving, IoT data ingestion, analytics pipelines | Application log monitoring, gaming analytics, web clickstreams | Fraud detection, anomaly detection, real-time dashboards, and streaming ETL workflows |
How AWS Kinesis works
AWS Kinesis operates as a real-time data streaming platform designed to handle massive amounts of data from various sources. The process begins with data producers—applications, IoT devices, or servers—sending data to Kinesis. Depending on the chosen service, Kinesis captures, processes, and routes the data in real time.
For example, Kinesis Data Streams breaks data into smaller units called shards, which ensure scalability and low-latency ingestion. Kinesis Firehose, on the other hand, automatically processes and delivers data to destinations like Amazon S3 or Redshift, transforming and compressing it along the way.
Users can access Kinesis through the AWS Management Console, SDKs, or APIs, enabling them to configure pipelines, monitor performance, and integrate with other AWS services. Kinesis supports seamless integration with AWS Glue, Lambda, and CloudWatch, making it a powerful tool for building end-to-end data workflows. Its serverless architecture eliminates the need to manage infrastructure, allowing businesses to focus on extracting insights and building data-driven applications.
Security
Security is a top priority for AWS, and Kinesis strengthens this by providing encryption both at rest and in transit, along with role-based access control to ensure data privacy. Furthermore, users can enhance security by enabling VPC endpoints when accessing Kinesis from within their virtual private cloud.
Kinesis offers robust features, including automatic scaling, which dynamically adjusts resources based on data volume to minimize costs and ensure high availability. Furthermore, it supports enhanced fan-out for real-time streaming applications, providing low latency and high throughput.
Video Streams
What it is:
Amazon Video Streams offers users an easy method to stream video from various connected devices to AWS. Whether it’s machine learning, playback, or analytics, Video Streams will automatically scale the infrastructure from streaming data and then encrypt, store, and index the video data. This enables live, on-demand viewing. The process allows integrations with libraries such as OpenCV, TensorFlow, and Apache MxNet.
How it works:
The Amazon Video Streams starts with the use of the AWS Management Console. After installing Kinesis Video Streams on a device, users can stream media to AWS for analytics, playback, and storage. The Video Streams features a specific platform for streaming video from devices with cameras to Amazon Web Services. This includes internet video streaming or storing security footage. This platform also offers WebRTC support and connecting devices that use the Application Programming Interface.
Data consumers:
MxNet, HLS-based media playback, Amazon SageMaker, Amazon Rekognition
Benefits:
- There are no minimum fees or upfront commitments.
- Users only pay for what they use.
- Users can stream video from literally millions of different devices.
- Users can build video-enabled apps with real-time computer-assisted vision capabilities.
- Users can playback recorded and live video streams.
- Users can extract images for machine learning applications.
- Users can enjoy searchable and durable storage.
- There is no infrastructure to manage.
Use cases:
- Users can engage in peer-to-peer media streaming.
- Users can engage in video chat, video processing, and video-related AI/ML.
- Smart homes can use Video Streams to stream live audio and video from devices such as baby monitors, doorbells, and various home surveillance systems.
- Users can enjoy real-time interaction when talking with a person at the door.
- Users can control, from their mobile phones, a robot vacuum.
- Secure Video Streams provides access to streams using Access Management (IAM) and AWS Identity.
- City governments can use Video Streams to securely store and analyze large amounts of video data from cameras at traffic lights and other public venues.
- An Amber Alert system is a specific example of using Video Streams.
- Industrial uses include using Video Streams to collect time-coded data such as LIDAR and RADAR signals.
- Video Streams are also helpful for extracting and analyzing data from various industrial equipment and using it for predictive maintenance and even predicting the lifetime of a particular part.
Data firehose
What it is:
Data Firehose is a service that can extract, capture, transform, and deliver streaming data to analytic services and data lakes. Data Firehose can take raw streaming data and convert it into various formats, including Apache Parquet. Users can select a destination, create a delivery stream, and start streaming in real-time in only a few steps.
How it works:
Data Firehose allows users to connect with potentially dozens of fully integrated AWS services and streaming destinations. The Firehose is basically a steady stream of all of a user’s available data and can deliver data constantly as updated data comes in. The amount of data coming through may increase substantially or just trickle through. All data continues to make its way through, crunching until it’s ready for visualizing, graphing, or publishing. Data Firehose loads data onto Amazon Web Services while transforming the data into Cloud services that are basically in use for analytical purposes.
Data consumers:
Consumers include Splunk, MongoDB, Amazon Redshift, Amazon Elasticsearch, Amazon S3, and generic HTTP endpoints.
Benefits:
- Users can pay as they go and only pay for the data they transmit.
- Data Firehose offers easy launch and configurations.
- Users can convert data into specific formats for analysis without processing pipelines.
- The user can specify the size of a batch and control the speed for uploading data.
- After launching, the delivery streams provide elastic scaling.
- Firehose can support data formats like Apache ORC and Apache Parquet.
- Before storing, Firehose can convert data formats from JSON to ORC formats or Parquet. This saves on analytics and storage costs.
- Users can deliver their partitioned data to S3 using dynamically defined or static keys. Data Firehose will group data by different keys.
- Data Firehose automatically applies various functions to all input data records and loads transformed data to each destination.
- Data Firehose gives users the option to encrypt data automatically after uploading. Users can specifically appoint an AWS Key Management encryption key.
- Data Firehose features a variety of metrics that are found through the console and Amazon CloudWatch. Users can implement these metrics to monitor their delivery streams and modify destinations.
Use cases:
- Users can build machine learning streaming applications. This can help users predict inference endpoints and analyze data.
- Data Firehose provides support for a variety of data destinations. A few it currently supports include Amazon Redshift, Amazon S3, MongoDB, Splunk, Amazon OpenSearch Service, and HTTP endpoints.
- Users can monitor network security with Event Management (SIEM) tools and supported Security Information.
- Firehose supports compression algorithms such as Zip, Snappy, GZip, and Hadoop-Compatible Snappy.
- Users can monitor in real-time IoT analytics.
- Users can create Clickstream sessions and create log analytics solutions.
- Firehose provides several security features.
Data streams
What it is:
Data Streams is a real-time streaming service that provides durability and scalability and can continuously capture gigabytes from hundreds of thousands of different sources. Users can collect log events from their servers and various mobile deployments. This particular platform puts a strong emphasis on security. Data streams allow users to encrypt sensitive data with AWS KMS master keys and a server-side encryption system. With the Kinesis Producer Library, users can easily create Data Streams.
How it works:
Users can create Kinesis Data Streams applications and other types of data processing applications with Data Streams. Users can also send their processed records to dashboards and then use them when generating alerts, changing advertising strategies, and changing pricing.
Data consumers:
Amazon EC2, Amazon EMR, AWS Lambda, and Kinesis Data Analytics
Kinesis Data Streams provides unmatched control over real-time data ingestion and scalability, ensuring low-latency performance for critical applications.
Benefits:
- Data Streams provide real-time data aggregation after loading the aggregate data into a map-reduce cluster or data warehouse.
- Kinesis Data Streams feature a delay time between when records are put in the stream and when users can retrieve them, which is approximately less than a second.
- Data Streams applications can consume data from the stream almost instantly after adding the data.
- Data Streams allow users to scale up or down, so users never lose any data before expiration.
- The Client Library supports fault-tolerant data consumption and offers support for scaling support Data Streams applications.
Use cases:
- Data Streams can work with IT infrastructure log data, market data feeds, web clickstream data, application logs, and social media.
- Data Streams provides application logs and a push system that features processing in only seconds. This also prevents losing log data even if the application or front-end server fails.
- Users don’t batch data on servers before submitting it for intake. This accelerates the data intake.
- Users don’t have to wait to receive batches of data but can work on metrics and application logs as the data is streaming in.
- Users can analyze site usability engagement while multiple Data Streams applications run parallel.
- Gaming companies can feed data into their gaming platform.
Data analytics
What it is:
Data Analytics provides open-source libraries such as AWS service integrations, AWS SDK, Apache Beam, Apache Zeppelin, and Apache Flink. It’s for transforming and analyzing streaming data in real time.
How it works:
Its primary function is to serve as a tracking and analytics platform. It can specifically set up goals, run fast analyses, add tracking codes to various sites, and track events. It’s important to distinguish Data Analytics from Data Studio. Data Studio can access a lot of the same data as Data Analytics but displays site traffic in different ways. Data Studio can help users share their data with others who are perhaps less technical and don’t understand analytics well.
Data consumers:
Results are sent to a Lambda function, Kinesis Data Firehose delivery stream, or another Kinesis stream.
Benefits:
- Users can deliver their streaming data in a matter of seconds. They can develop applications that deliver the data to a variety of services.
- Users can enjoy advanced integration capabilities that include over 10 Apache Flink connectors and even the ability to put together custom integrations.
- With just a few lines of code, users can modify integration abilities and provide advanced functionality.
- With Apache Flink primitives, users can build integrations that enable reading and writing from sockets, directories, files, or various other sources from the internet.
Use cases:
- Data Analytics is compatible with the AWS Glue Schema Registry. It’s serverless and lets users control and validate streaming data while using Apache Avro schemes. This is at no additional charge.
- Data Analytics features APIs in Python, SQL, Scala, and Java. These offer specialization for various use cases, such as streaming ETL, stateful event processing, and real-time analytics.
- Users can deliver data to the following and implement Data Analytics libraries for Amazon Simple Storage Service, Amazon OpenSearch Service, Amazon DynamoDB, AWS Glue Schema Registry, Amazon CloudWatch, and Amazon Managed Streaming for Apache Kafka.
- Users can enjoy “Exactly Once Processing.” This involves using Apache Flink to build applications in which processed records affect results. Even if there are disruptions, such as internal service maintenance, the data will still process without any duplicate data.
- Users can also integrate with the AWS Glue Data Catalog store. This allows users to search multiple AWS datasets
- Data Analytics provides the schema editor to find and edit input data structure. The system will recognize standard data formats like CSV and JSON automatically. The editor is easy to use, infers the data structure, and aids users in further refinement.
- Data Analytics can integrate with both Amazon Kinesis Data Firehose and Data Streams. Pointing data analytics at the input stream will cause it to automatically read, parse, and make the data available for processing.
- Data Analytics allows for advanced processing functions that include top-K analysis and anomaly detection on the streaming data.
AWS Kinesis vs. Apache Kafka
In data streaming solutions, AWS Kinesis and Apache Kafka are top contenders, valued for their strong real-time data processing capabilities. Choosing the right solution can be challenging, especially for newcomers. In this section, we will dive deep into the features and functionalities of both AWS Kinesis and Apache Kafka to help you make an informed decision.
Operation
AWS Kinesis, a fully managed service by Amazon Web Services, lets users collect, process, and analyze real-time streaming data at scale. It includes Kinesis Data Streams, Kinesis Data Firehose, and Kinesis Data Analytics. Conversely, Apache Kafka, an open-source distributed streaming platform, is built for real-time data pipelines and streaming applications, offering a highly available and scalable messaging infrastructure for efficiently handling large real-time data volumes.
Architecture
AWS Kinesis and Apache Kafka differ in architecture. Kinesis is a managed service with AWS handling the infrastructure, while Kafka requires users to set up and maintain their own clusters.
Kinesis Data Streams segments data into multiple streams via sharding, allowing each shard to process data independently. This supports horizontal scaling by adding shards to handle more data. Kinesis Data Firehose efficiently delivers streaming data to destinations like Amazon S3 or Redshift. Meanwhile, Kinesis Data Analytics offers real-time data analysis using SQL queries.
Kafka functions on a publish-subscribe model, whereby producers send records to topics, and consumers retrieve them. It utilizes a partitioning strategy, similar to sharding in Kinesis, to distribute data across multiple brokers, thereby enhancing scalability and fault tolerance.
What are the main differences between data firehose and data streams?
One of the primary differences is in each building’s architecture. For example, data enters through Kinesis Data Streams, which is, at the most basic level, a group of shards. Each shard has its own sequence of data records. Firehose delivery stream assists in IT automation, by sending data to specific destinations such as S3, Redshift, or Splunk.
The primary objectives between the two are also different. Data Streams is basically a low latency service and ingesting at scale. Firehose is generally a data transfer and loading service. Data Firehose is constantly loading data to the destinations users choose, while Streams generally ingests and stores the data for processing. Firehose will store data for analytics while Streams builds customized, real-time applications.
Detailed comparisons: Data Streams vs. Firehose
AWS Kinesis Data Streams and Kinesis Data Firehose are designed for different data streaming needs, with key architectural differences. Data Streams uses shards to ingest, store, and process data in real time, providing fine-grained control over scaling and latency. This makes it ideal for low-latency use cases, such as application log processing or real-time analytics. In contrast, Firehose automates data delivery to destinations like Amazon S3, Redshift, or Elasticsearch, handling data transformation and compression without requiring the user to manage shards or infrastructure.
While Data Streams is suited for scenarios that demand custom processing logic and real-time data applications, Firehose is best for bulk data delivery and analytics workflows. For example, Firehose is often used for IoT data ingestion or log file archiving, where data needs to be transformed and loaded into a storage or analytics service. Data Streams, on the other hand, supports applications that need immediate data access, such as monitoring dashboards or gaming platform analytics. Together, these services offer flexibility depending on your real-time streaming and processing needs.
Why choose LogicMonitor?
LogicMonitor provides advanced monitoring for AWS Kinesis, enabling IT teams to track critical metrics and optimize real-time data streams. By integrating seamlessly with AWS and CloudWatch APIs, LogicMonitor offers out-of-the-box LogicModules to monitor essential performance metrics, including throughput, shard utilization, error rates, and latency. These metrics are easily accessible through customizable dashboards, providing a unified view of infrastructure performance.
With LogicMonitor, IT teams can troubleshoot issues quickly by identifying anomalies in metrics like latency and error rates. Shard utilization insights allow for dynamic scaling, optimizing resource allocation and reducing costs. Additionally, proactive alerts ensure that potential issues are addressed before they impact operations, keeping data pipelines running smoothly.
By correlating Kinesis metrics with data from on-premises and other cloud performance services, LogicMonitor delivers holistic observability. This comprehensive view enables IT teams to maintain efficient, reliable, and scalable Kinesis deployments, ensuring seamless real-time data streaming and analytics.
Subscribe to our blog
Get articles like this delivered straight to your inbox