You may be using your log data in a completely wrong way. Today, your business produces more data than ever before, and log data is at the center of all this because it contains the signals of what caused a problem. If your teams have to search for these signals in an ad-hoc manner, then they are wasting their precious time. Nearly every company in existence is dealing with this challenge because it may not have the tools to filter these signals from the noise.
If you aren’t able to sort through all of your logs due to sheer volume, some folks choose to ignore them. If your monitoring tools are not helping you save valuable time in troubleshooting problems, you have the wrong tools. The hidden anomalies in your logs can help explain why incidents occur and can help prevent future disruptions. You just need to find a way to get your logs working for you, intelligently.
The answers lie within your data… somewhere
Every new device that is added, and every new code release that is pushed, contributes to log overload. These form part of what is called “machine data”, which is growing 50x faster than traditional business data. In fact, everything in your stack is continuously writing new events to your log files.
The good news is that log data can be hugely valuable for fast-moving organizations because it contains the behavior patterns of your applications and infrastructure. However, discerning which data is relevant can often be too big of a challenge for even the most experienced teams. This is why LogicMonitor believes in taking an algorithmic approach to logs.
Anomaly detection automates relevant data discovery
99.999% of machine data produced is repetitive and does not require human attention. This is the data that is produced when everything is “business as usual.” And it’s not the data that will help you troubleshoot faster. In fact, it will just slow you down. It’s understanding the unknown changes that will expedite your troubleshooting process. At that point in time, you need the fastest way to know what changed within your environment, and why it changed.
At LogicMonitor, we believe that using machine learning is the best way to automatically parse through repetitive data and determine what actually requires the attention of a skilled expert on your team. Anomaly detection uses machine learning to identify changes in expected patterns of behavior. By continuously monitoring a live environment, anomaly detection algorithms can effectively expose themselves to enormous amounts of live training data. This allows them to understand what is business as usual and what data is an outlier for an organization’s specific environment.
Since all environments are typically in a constant state of change, effective anomaly detection algorithms must continuously learn, rather than rely on heuristic models. If they don’t continuously learn, they risk missing subtle parameter changes, or can produce false positives.
Like any kind of machine-learning-based approach, the more data that is made available to the system, the more accurate the system becomes. This is one of the most important aspects of anomaly detection in general. The largest risks that your organization will encounter will almost certainly be unknown, or in other words, something you didn’t prepare for in advance. You need your system to be as experienced and accurate as possible in order to detect a new unknown that signals the risk. Using anomaly detection can prevent, or at the very least, catch new or unknown issues before they negatively impact your business.
The future of anomaly detection is proactive, not reactive
Disruptions happen due to changes and they may cause severe outages impacting your business. But if you can see the changes that are happening in your environment in near real-time, you can prevent these disruptions, and thus the outages. In today’s digital business environment where a significant portion of the business runs through applications, being reactive to outages isn’t an option anymore.
Take a bank, for example, that doesn’t know what every high-risk incident will look like. In this case, it’s impossible for the bank to search in advance for all incidents, write rules to identify anomalous data, or even build statistical models to prevent it. Only with a machine learning approach that adapts to the continuous change can safeguard against future unknown IT issues.
LogicMonitor has offered Anomaly Detection for metrics for quite some time already, but up until now, we haven’t specialized in logs. In Q4 2020, I’m proud to announce that we will be making Anomaly Detection for logs available to all of our customers in the form of our newest product, LM Logs™. Our groundbreaking algorithmic approach to logs will make it easier for you and your teams to filter signals from the noise, and solve problems faster than ever before.
LM Logs is coming soon. In the meantime, if you’re interested in joining our LM Logs beta, please click here to express interest in shaping the future of IT alongside us.
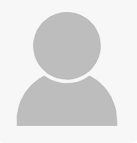
Subscribe to our blog
Get articles like this delivered straight to your inbox